Using AI to help solve Bloom’s Two Sigma Problem
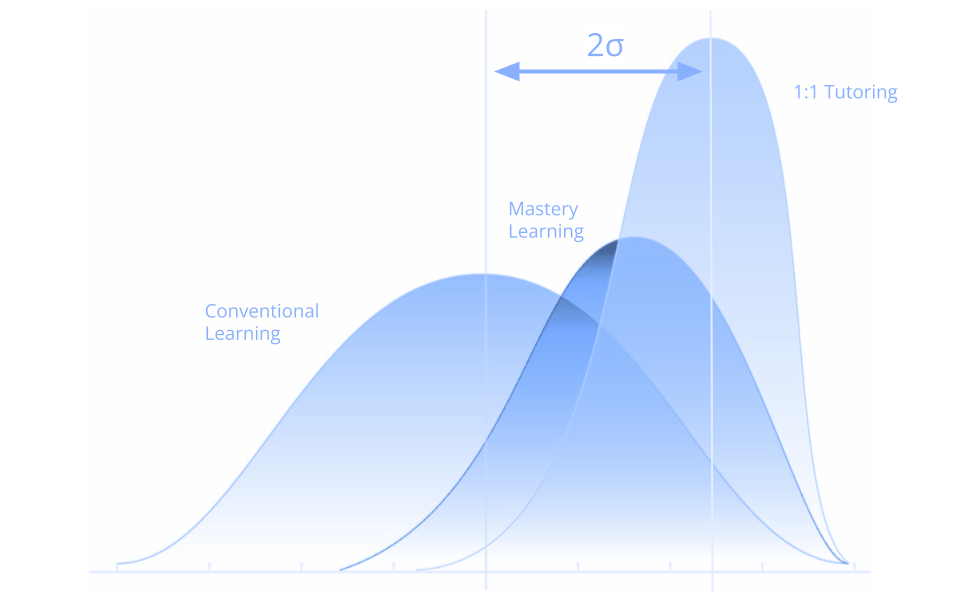
Imagine we’re all surfers. The ocean we’re in is the educational system, and we’re all trying to ride the wave of knowledge to the shore of understanding. Some of us have master surfers as guides – personal tutors who are right there with us, helping us manoeuvre the currents and ride high on the knowledge wave. They know our strengths, they know our fears, and they ensure we don’t wipe out. These fortunate few reach the shore faster, more smoothly and often with a lot more fun.
Then there are the rest of us. We’re in a giant surf class. There’s one instructor and dozens of us learners. The instructor is doing their best, but they can’t give us all the personalised attention we need. Some of us catch the wave, some of us don’t. This is Bloom’s Two Sigma Problem.
Brought to the fore by educational psychologist Benjamin Bloom in the 1980s, the Two Sigma Problem highlights a gap in education. Personal tutoring can propel students’ performance by two standard deviations – like moving from the middle of a class right to the top 2%. The problem is, we can’t give everyone a personal tutor. It’s just not feasible. So, the question is, how do we give each student the benefits of one-on-one instruction, at scale?
Enter Artificial Intelligence (AI) and, in particular, Large Language Models (LLMs) such as ChatGPT. I’ve been experimenting with using ChatGPT as a tutor for my son during the revision period for his exams. It’s great at coming up with questions, marking them, and suggesting how to improve. This kind of feedback is absolutely crucial to learning. It’s also great at exploring the world and allowing curiosity to take you in new directions.
So, if we revisit the Two Sigma Problem based on what’s possible with LLMs, it looks like there’s a possible solution with multiple advantages:
- Personalisation: Like a master surfer guiding us through the waves, AI offers individualised instruction. It can adapt to each learner’s pace, skill level, and areas of interest. It’s like your own personal Mr. Miyagi, providing the right lesson at the right time. Wax on, wax off.
- 24/7 Availability: With AI, it’s always high tide. The learning doesn’t stop when the school bell rings. Whether it’s the middle of the day or the middle of the night, your AI tutor is there to help, guide, and explain.
- Scalability: One-to-one tutoring might not be feasible, but AI makes one-to-one-to-many a reality. An AI tutor doesn’t get exhausted or overbooked. It can help an unlimited number of students at once, ensuring everyone gets the ride of their lives on the knowledge wave.
- Feedback and Assessment: Picture a surf instructor who can instantly replay your wipeouts, showing you exactly what went wrong and how to fix it. That’s what AI can do. It provides immediate feedback, helping learners understand and correct their mistakes right away.
- Enhanced Resources: LLMs are like a treasure trove of knowledge. Trained on a vast array of educational content, they’re like having the British Library at your fingertips, ready to generate explanations, examples, and answers on a multitude of topics.
- Removing Bias: AI doesn’t care about your background, your accent, or the colour of your board shorts. When designed and trained properly, it treats all learners equally, providing a level playing field.
No technology is a silver bullet. As an educator, I know that while curiosity and feedback is really important, there’s nothing like another human providing emotional input — including motivation. AI is here to support, not replace, our human guides.
Even though it’s early days, we’re already seeing some really interesting developments in the application of LLMs in education. I’m no fan of Microsoft, but I will acknowledge that a feature they have in development called ‘passage generation’ looks interesting. This tool reviews data to create personalised reading passages based on the words or phonics rules a student finds most challenging. Educators can customise the passage, selecting suggested practice words and generating options, then publish the passage as a new reading assignment. I find this kind of thing really useful in Duolingo for learning Spanish. Context matters.
As a former teacher, I know how important prioritisation can be for the limited amount of time you have with each student. And as a parent, I’m a big believer in the power of deliberate practice for getting better at all kinds of things. Freeing up teachers to be more like coaches than instructors has been the dream ever since someone came up with the pithy phrase “guide on the side, not sage on the stage”.
One of the main concerns I think a lot people have with AI in general is that it will “steal our jobs”. I’d point out here that the main problem here isn’t AI, it’s capitalism. Any tool or system be used for good or for ill. If you’re not sure how we can approach this post-scarcity world, I’d recommend reading Fully Automated Luxury Communism by Aaron Bastani. Of course, regulation is and should be an issue, too.
The main issue I see with this is centralised LLMs run by companies running opaque models and beholden to shareholders. That’s why I envisage educational institutions running local LLMs, or at least within a network that only connects to the internet when it needs to. Just as Google Desktop used to allow you to search through your local machine and the web, I can imagine us all having an AI assistant that has full context, while preserving our privacy.
So the way to approach any new tool or service is to ask critical questions such as “who benefits?” but also to fully explore what’s possible with all of this. I’m hugely hopeful that AI won’t lead us into a sci-fi dystopia, but rather help to even out the playing field when it comes to human learning and flourishing.
What do you think? I’d love to hear in the comments!
Image remixed from an original on the SkillUp blog. Text written with the help of ChatGPT (it’s particularly good at coming up with metaphors, I’ve found!)